FinTech Case Studies
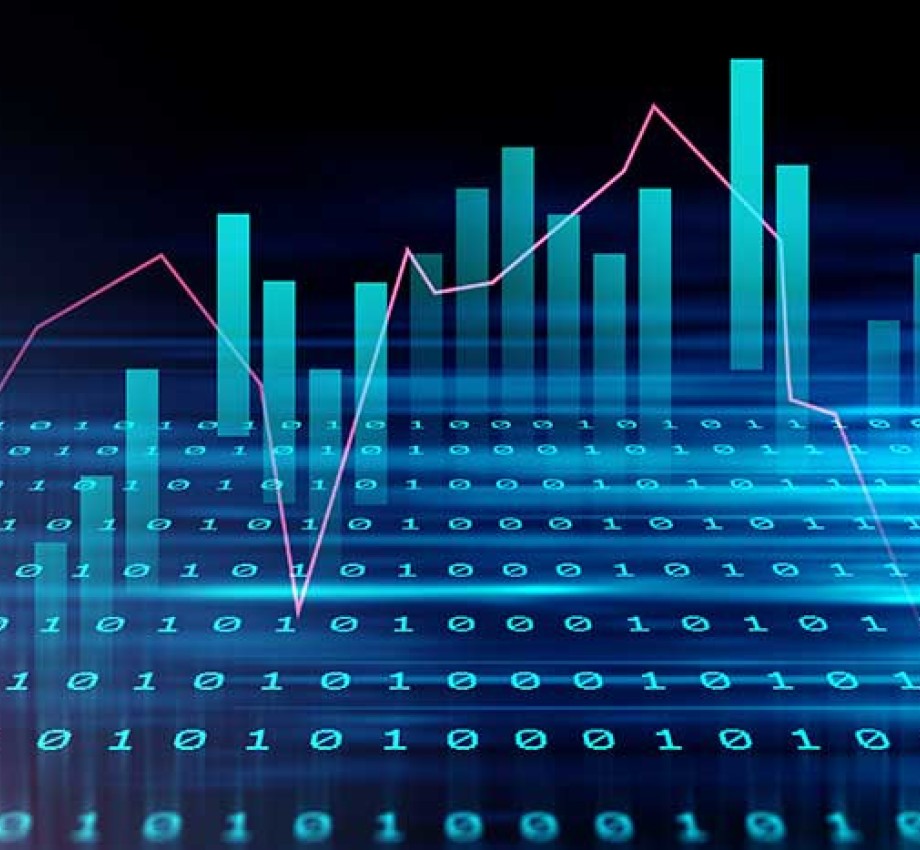
UC Berkeley Haas Global Access Program (BHGAP) and BHGAP Innovation Program faculty member Gregory La Blanc recently hosted a Zoom meeting where he gave viewers a sneak peek into his FinTech class.
Check out the highlights from his talk below and watch the full video!
Citibank was the 300th largest bank in the United States. How do you go from the 300th largest bank in the United States to the largest credit card issuer in the entire world?
With machine learning, with data science, with analytics.
They saw that every single bank in the country is using the same scoring model: If your credit score is above this cutoff, you get a loan; if you're below this cutoff, you don't get a loan.
They said that there must be a more fine-grained model—to find the people who are rejected and see if a few of them might turn out to be good customers. They lent a whole bunch of money to people whom the other model said don't lend money to.
There were a lot of defaults. A lot of people didn't repay their loans. There was a reason why they were generally considered bad credits.
However, after seven or eight months, they were able to determine that a small percentage of them were good credits. So after seven or eight months, they ended the experiment. They cancelled the credit cards of most of the customers.
And what were they left with? A couple of good customers and an amazing proprietary database. They had some training data that they could then feed through an algorithm, and that algorithm would say, here is what makes these good customers similar to one another, and here is what makes bad customers similar to one other. So if you want to find good credit risks, go look for people who look like this.
That's how they were able to scale and grow and become the largest credit card issuer in the world.
This is what's given rise to lots of new startups in the borrowing and lending space. All of them are using different types of machine learning models to identify good credit risks.
In my class, we also hear from people who work at peer-to-peer lending companies. What they really should be called are algorithmic lenders. They use data that other banks don't use. They're going beyond the traditional data sets and finding signals of credit-worthiness that the traditional banks don't look at.
One thing they could look at is your location data.
If a bank is tracking my location, they know something about me. They know that I went to work. Then I spent a couple of hours at LinkedIn headquarters. Then I head over to Google headquarters and then I hit Facebook headquarters for a couple hours in the afternoon.
Banks can use this information to revise my credit score up or down.
If we drill down into location data, we can figure out whom this person is meeting with. What room are they in inside the Facebook headquarters? What building are they in? Who are they sitting next to at that meeting?
If you don't have a unique, proprietary data source, you don't have a sustainable competitive advantage and you'll ultimately be commodified.
There's a company called Tala, and it works in countries like Kenya where people have no documentation: no banking history, no income history, no employment history, no housing history. And yet they can still lend money to these people because they download the Tala app, and the app scours their phone for data.
Kai-Fu Lee, a famous Chinese venture capitalist, visited Haas about two years ago, and he talked about a FinTech startup that he'd invested in. This startup used alternative data to evaluate credit. And he said that one of the most important signals that they had discovered of credit-worthiness was how people managed their battery life. You can track this because you have access to their phone data. People who let their batteries go all the way down to zero are bad credit risks compared to those who are topping off their batteries at 20 or 30 percent.
Even though it's absolutely essential and critical that every company use analytics, machine learning and data science, that alone cannot provide you with a competitive advantage. At the end of the day, that is a commodity. Anybody can learn how to do this, and anybody can hire away anybody else who knows how to do this.
Your competitive advantage has to come from your data—not from what you do with the data or how you use the data. I would argue that if you don't have a unique, proprietary data source, you don't have a sustainable competitive advantage and you'll ultimately be commodified.